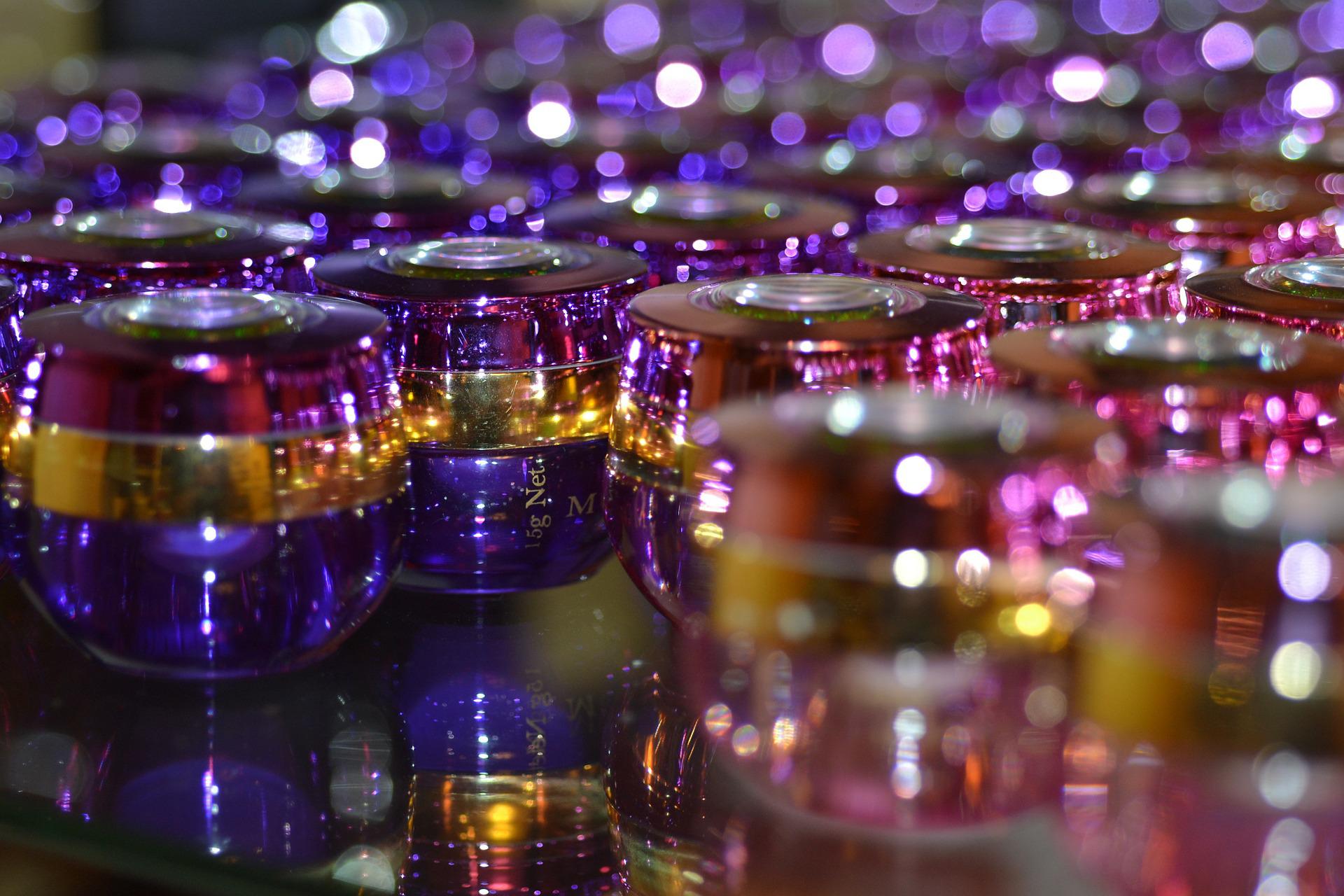
Evaluating the skin sensitisation potential of a squaric acid
The SaferSkin™ Sensitization application implements three well-adopted defined approaches to perform an integrated analysis of the OECD-approved in vitro tests (DPRA, KeratinoSens™, h-CLAT) for assessment of skin sensitisation potential. The approach may be further extended by additional in silico and in vitro methods to strengthen the weight of evidence in an ingredient or formulation assessment. Here we concentrate on the current core OECD-approved tests.
The three defined approaches developed at P&G, Givaudan, and BASF, each with its own pros and cons, for the integrated analysis are:
- Bayesian Network
- Multiple Linear Regression
- “2-out-of-3” Weight of Evidence
While the last simplest method can be used for hazard assessment only (sensitiser/non-sensitiser), the first two more advanced and complex approaches (Bayesian Network and Multiple Linear Regression), can be adopted for risk assessment, as they are able to predict the compound’s potential for skin sensitisation. In particular, they are able to predict the compound’s pEC3 value in the in vivo LLNA test, which is traditionally used for further risk assessment and safe-dose evaluation.
Let us have a look at how the SaferSkin use for sensitization looks in practice. Imagine we would like to evaluate a skin sensitisation potential of a squaric acid for which we performed classical in vitro tests with the following results:
The results of in vitro tests can be easily provided to the SaferSkin platform through a user-friendly interface:
By a simple click on the “Make a prediction” button, the results of the three defined approaches are summarized:
In this case, the most complex approach (the Bayesian Network) predicted with substantial confidence that the compound is a moderate sensitiser. The other two approaches, Multiple Linear Regression and “2 out of 3” Weight of Evidence, which is not able to provide confidence, predicted the compound to be non-sensitiser. In this particular case, we know the actual result of the in vivo LLNA test, where the compound was determined to be a moderate sensitiser.
The reason for the misprediction of the simpler two approaches lies in the fact that two out of three in vitro tests were negative, indicating that the compound is a non-sensitiser. However, the Bayesian network approach, which takes into account also the in silico descriptors based on the compound’s structure was able to better weigh the evidence by decreasing the importance of negative in vitro test results on the account of in silico predictions. The model learned how to weigh the evidence in different situations during the training phase where the parameters of the Bayesian network were fitted on the set of 144 compounds with complete data. The reader can take this example as a cautionary warning, as they could have decided to use the simpler voting approach, and although they would use OECD assay data and a defined approach, they would obtain a completely incorrect answer, and with no warning provided by the method.
We can also see a more detailed report for each of the three defined approaches, where we can see that the Bayesian Network integrated approach predicted a pEC3 value of -1.0, which is exactly the same as the actual in vivo result in the LLNA test.